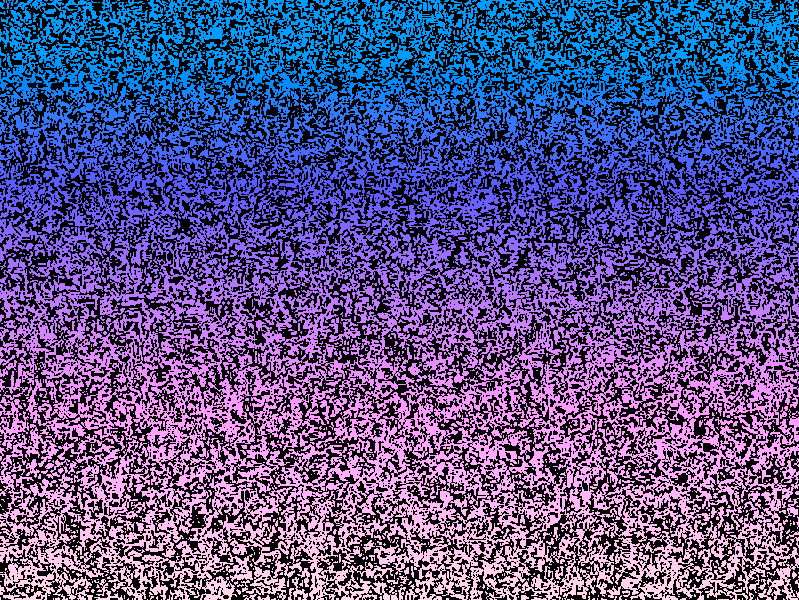
Highlights §
- There are a gajillion articles about ‘the modern data stack’ and ‘data warehouse good’ and ‘how to do data modeling’, and not a single one about what to do when you look at a chart. (View Highlight)
- When you open a business dashboard in your company, do you feel a little confused? Like you don’t know what to do? I mean, look at this chart [he shows a wiggly line, with no clear pattern]. What can you conclude from it? Is the metric doing well? Is it going up overall? Is it business as usual? Or are things getting worse? Should you be worried? (View Highlight)
- • at how things are going.
• Figure out when things were going better or worse than usual.
• Hypothesize why that might’ve happened.
• Do more of the potentially good things and less of the potentially bad things.
• See if things get better again.
• Learn how things work (View Highlight)
- On the other hand, there’s something profound about Chin’s post. Despite having read hundreds of Medium articles and VC blogs about how about which product adoption metrics are best, or how to understand user cohorts, or how to benchmark your company’s KPIs, it was Chin’s post, and only Chin’s post, that inspired me to immediately dump a bunch of Substack metrics into a spreadsheet and see they taught me.5 Why, despite the underlying theory being simple, obvious, and something I already do informally every day, did Chin’s articulation of it land so differently? (View Highlight)
- One answer is packaging—it’s an engaging read; he prescribes a way to find anomalies, called XmR charts, that have a satisfying visual aesthetic; the social proof from Amazon, and from the depth of Chin’s own research, is hard to ignore. But none of those things are that unique. Every data conference is full of glossy presentations about how some amazing company built itself, and we often start forgetting those talks before they’re even over. (View Highlight)
- The better answer, I think, is that Chin’s post gives us permission to make this simple. When we look across a sea of data, it’s easy to be overwhelmed by the difficulty and depth of it all. It’s easy to assume that there are so many complications; so much confounding; so many numbers; so little time. When working in this sort of environment, we often play up to the complexity of our competition—by using candlestick charts, or fancy math, or formal theories of causal inference, or our time-honored tradition of smugly saying, “well, you see, it depends.” (View Highlight)
- Chin tells us to throw all of that out. He’s studied this more than we have, and has talked to people who made this work better than we did. And he says it’s okay to draw some trend lines, to kinda eyeball some error bars, and to not fret too much about the details. The power of this instruction isn’t in what it tells us to do—most of us are already pretty accustomed to looking at dashboards and trying to figure out why it went up and down—but in what it tells us not to do. Don’t try to solve your problems in a novel way, he says; try just drawing these charts first. Don’t worry about those wiggles, unless they persist for too long or get too severe. Don’t worry about the precise methods for detecting anomalies; use this simple formula.6 (View Highlight)
- As an analyst, an executive, a day trader, or even a blogger starting at a few Substack dashboards, every chart is a temptation—surely, you think, if you look at it at just the right angle, it will reveal its secrets. This is why casinos, which control every input on their gambling floors, put dashboards on roulette wheels—not to inform us, but to bait our natural inclinations to see patterns in every chart. (View Highlight)
- Chin reminds us of the danger of this approach. When you investigate everything, you find nothing; when you find nothing, you doubt your methods; and when you doubt your methods, you flail: (View Highlight)
- But we already know how to aim: Look for variation, ask what caused it, repeat. We don’t need to teach ourselves how to do this; we just need to be reminded—as Chin is reminding us, now—that those simple steps are enough. (View Highlight)