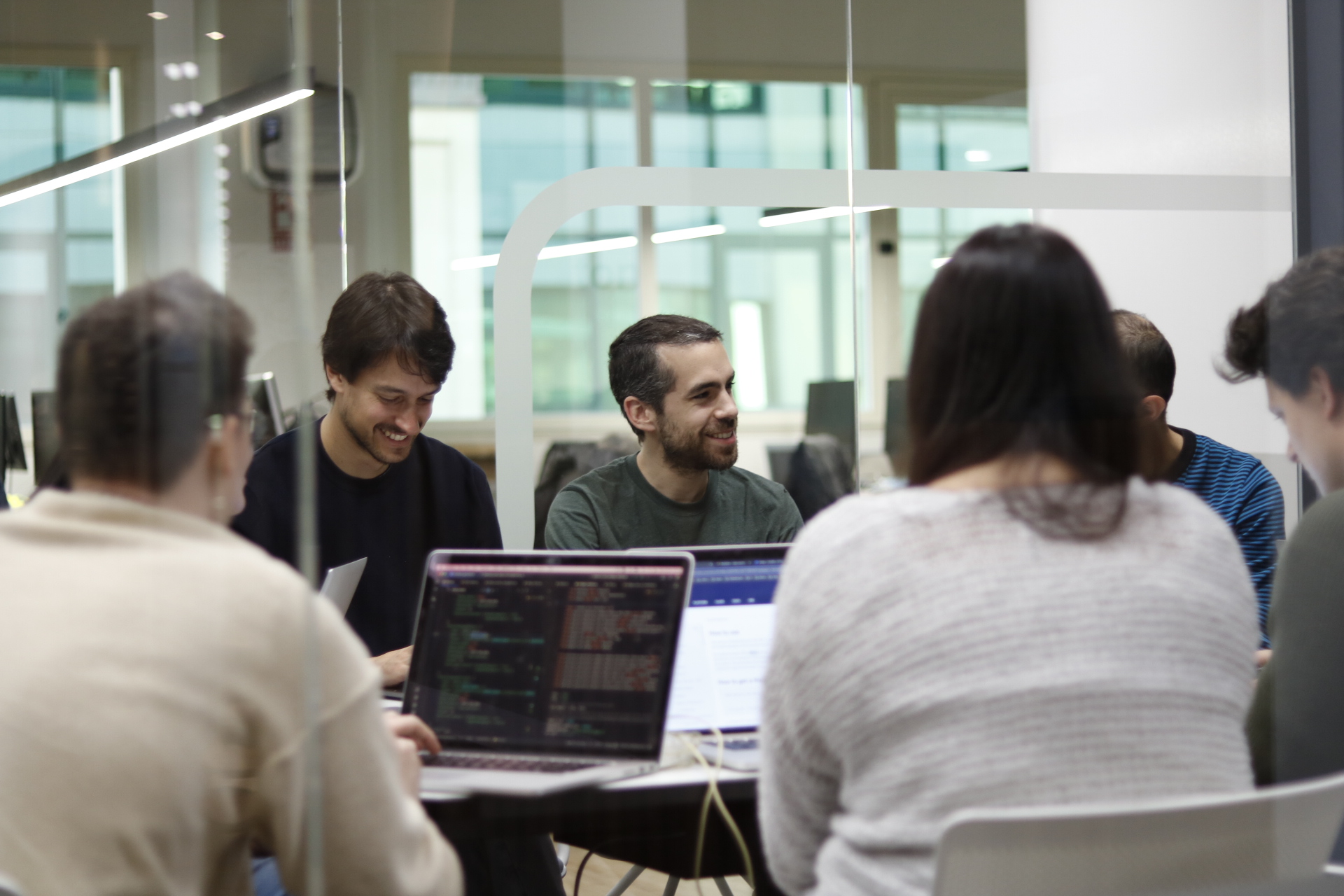
Highlights §
- . This reconfiguration brings together disciplines that were previously somewhat distant, such as business, design, data, and technology. Bringing all these profiles together and working together allows us to create better data-driven solutions that benefit other areas of the bank and our clients in the form of new products and services. (View Highlight)
- The teams or programs in which BBVA AI Factory participates are made up of experts in various disciplines, from data science, engineering, and software development to design and business. Experts in digital law, regulation, privacy, and security complement them. (View Highlight)
- In fact, the key to these AI teams lies in their multidisciplinary nature—that is, in their ability to coordinate different types of profiles under the same roof (or scrum) to drive a specific product forward. This is where the role of the Scrum Master comes into play. The Scrum Master facilitates the smooth flow of work, helps to overcome blockages, and ensures that team members work in sync. (View Highlight)
Figure 1. For example: a team or a scrum schema with a data component that is part of a specific business vertical. (View Highlight)
- There are several types of team structures. The fundamental difference is in the environment where the developed models will be run. For example, if a team develops models whose output is displayed in the bank’s mobile application, it becomes essential to integrate design profiles with expertise in user experience (UX) and software development (IT). On the other hand, if the models will be executed in the bank’s internal structures, it is essential to have people who can help transfer the information to these specific environments, such as profiles from BBVA’s engineering department. (View Highlight)
-
- Staying true to the purpose, not the task: an end-to-end perspective
When a team does not know the ultimate purpose for which it is working, it tends to focus on solving specific and unrelated tasks, neglecting to focus on achieving the overall goal.
In contrast, AI teams that share the same goal, focus on achieving it, and share responsibility for the project improve work quality: there are more eyes to catch mistakes. This approach also increases knowledge sharing and flexibility, improving workflow and time to market. Moreover, the end-to-end perspective increases the commitment of its members, who can see the ultimate impact of their work. (View Highlight)
To keep in mind: Potential disorganization
Synchronizing people from different teams under the same umbrella can be chaotic. Previously, tasks were organized in layers; now, tasks are planned in parallel with this scheme. It is essential to keep the end goal in mind without losing sight of everyone involved’s goals, uncertainties, and blockages. (View Highlight)
-
- More autonomy, more to learn
While multidisciplinary team members tend to have a clear understanding of their responsibilities and skills, they also have the opportunity to take on tasks they would not normally do. This promotes professional growth and allows everyone to benefit from other team members’ contributions. (View Highlight)
- o keep in mind: Frustrated self-management
In theory, team members can choose their tasks organically. However, unless teams are at a certain maturity level, tasks are likely to be assigned, which can frustrate team members by not allowing self-organization. Ideally, no specific tasks should be assigned. Instead, each person should be free to take on work that they feel is in line with their expertise and interests, depending on the goals. (View Highlight)
-
- Creating a common language and clear communication
One of the most significant challenges multidisciplinary teams face is encounters with people who speak a different language – professionally speaking. While this can be limiting, it is the perfect opportunity to ask questions and not assume others understand when we say “feature,” “cluster,” or “score.” These words have different connotations depending on the world in which you operate. Therefore, finding a common language and aligning our messages is critical to the success of a hybrid team. (View Highlight)
-
- Bridging the problem-solution gap
The increasing proximity of the business to the technical teams favors the creation of shared spaces that bridge the gap between the need and the possible solution. These spaces encourage dialog and reduce the time spent on inference that tends to occur when these areas are unfamiliar. (View Highlight)
- To keep in mind: Rejection of the unknown
There is still some resistance to change among non-technical people when it comes to using data and AI solutions. Therefore, it is essential to build trust in teams to allow them to reach their full potential. This will lead to better results over the medium and long term. Trust is earned incrementally by demonstrating the potential of data in tangible and practical ways. (View Highlight)
- In this context, several roles help with translating between the two worlds to ensure that data’s potential contributes to the advancement of business objectives. They are responsible for communicating the power of data and AI to our stakeholders while managing expectations, as implementing these types of solutions takes time, resources, and iterations. These are Product Owners (PO), Data Leads (DL), and Business Translators (BT). (View Highlight)
- The Business Translator is a specific role that, as the name suggests, is responsible for translating both business requirements and technical solutions. It is the profile that mediates between the two worlds.
The BT communicates the solution’s capabilities to stakeholders using an accessible narrative and as little technical jargon as possible. They can use analogies, demonstrations, references to other industries, or examples from experience. The idea is to translate something somewhat abstract, such as a data solution, into something tangible and easy to understand. When translating, it is important to be pragmatic in the linguistic sense: to adapt the message to suit the audience without losing essential details. (View Highlight)
New highlights added March 6, 2024 at 3:39 PM §
- Product Owners and Data Leads
POs and DLs have different backgrounds and come from different knowledge domains, either closer to the business or the technical world. In any case, they are hybrid profiles able to understand the various aspects of a project, such as business logic, data, technology, or privacy, among others. They have a strategic vision of the product and are concerned with integrating the skills and experience of the different roles in the team to maximize the value of the proposed solution.
The data design phase, which describes and blueprints the solution, is the first step when starting a new project. POs and DLs work with their teams to brainstorm different ideas for solving the problem. As soon as the business challenge is translated into a data-driven solution, the team gets to work. (View Highlight)
- Our teams include members from different disciplines because all these areas of knowledge are necessary to develop data-driven products. Moreover, different eyes see different things: they enhance the ability to understand problems from more perspectives and arrive at better solutions to achieve our goals.
Recent advances in AI, especially those related to generative AI, lead us toward including more humanistic profiles in work teams. These profiles will ultimately be more integrated into developing technology-based products and services. (View Highlight)